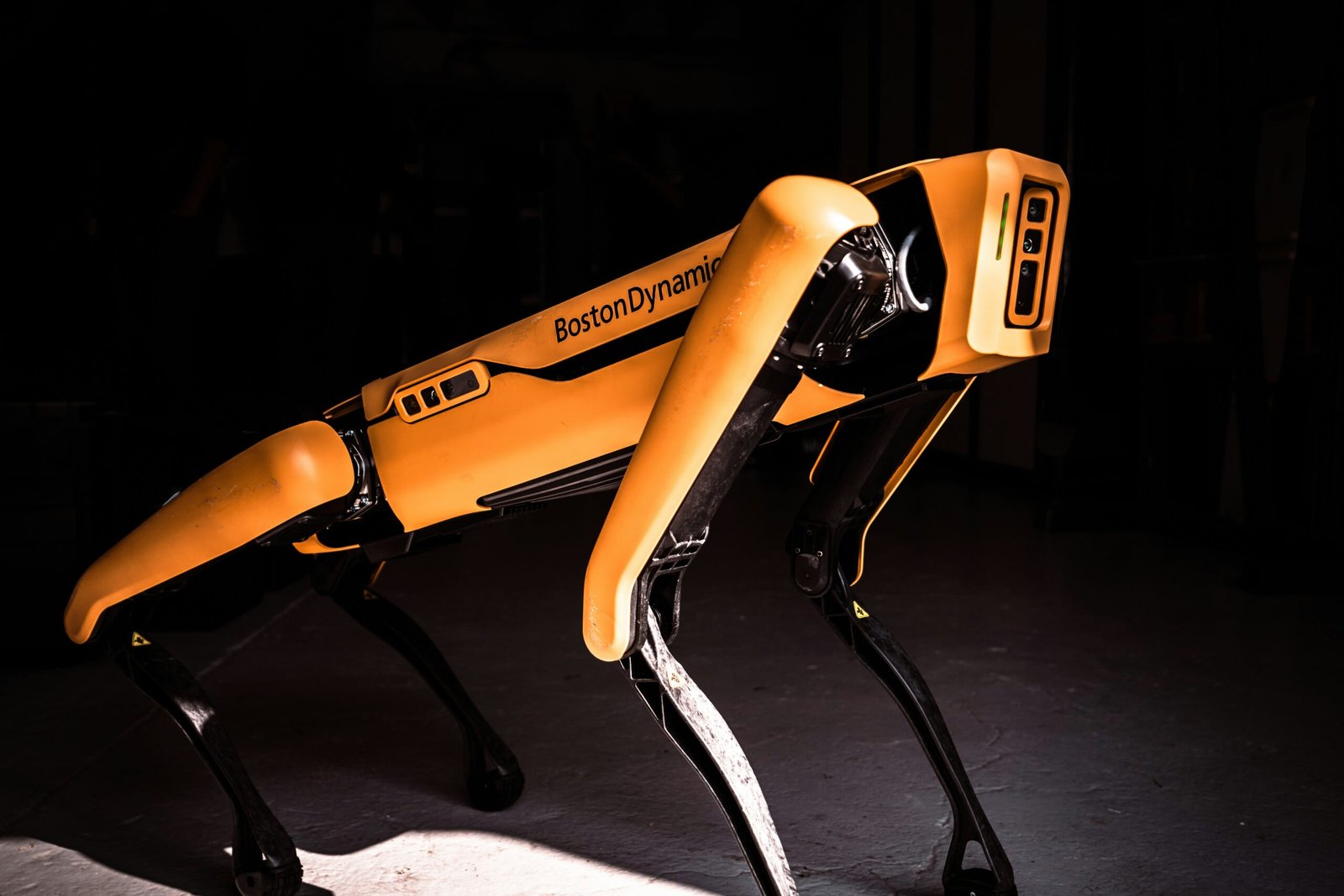
Introduction to Predictive Maintenance and Industry 4.0
Predictive maintenance has revolutionized the industry by shifting the paradigm from traditional reactive and preventive maintenance practices to a more foresighted approach. Traditional maintenance strategies often relied on scheduled checks or responded to equipment failures, which could lead to unexpected downtimes and higher costs. However, predictive maintenance leverages real-time data to anticipate and address potential issues before they escalate, thereby enhancing operational efficiency and extending the lifespan of machinery.
This evolution from preventive to predictive maintenance wouldn’t be possible without the advancements heralded by Industry 4.0. Often dubbed the Fourth Industrial Revolution, Industry 4.0 introduces an array of cutting-edge technologies such as the Internet of Things (IoT), artificial intelligence (AI), and big data, all of which play a pivotal role in enabling predictive maintenance.
IoT involves the interconnectivity of physical devices, allowing for seamless data exchange and continuous monitoring of equipment performance. By equipping machinery with IoT sensors, industrial facilities can collect granular data on parameters like temperature, vibration, and pressure in real-time. This data is then transmitted to analytics platforms where it can be processed and analyzed.
Artificial intelligence, particularly machine learning algorithms, is integral to making sense of the vast amounts of data collected. These algorithms can detect patterns, predict future conditions, and recommend maintenance actions based on historical data and real-time inputs. AI-driven predictive maintenance models can learn and adapt, continually improving their accuracy over time.
Big data analytics further enhances predictive maintenance by enabling the processing and analysis of large datasets to generate actionable insights. This helps in understanding complex dependencies and trends that might not be discernible through traditional methods. The integration of big data with IoT and AI provides a robust framework for predictive maintenance, making industrial processes more intelligent and efficient.
In essence, the convergence of IoT, AI, and big data within the Industry 4.0 framework empowers industries to adopt predictive maintenance, transforming maintenance operations from a cost center to a value generator. The digital twins concept, a digital replica of physical assets, also plays a significant role by allowing for simulation and analysis, further optimizing maintenance strategies.
Key Technologies Enabling Predictive Maintenance
Predictive maintenance has become a vital component of Industry 4.0, driven by an array of advanced technologies. Central to this transformation is the integration of Internet of Things (IoT) sensors, which gather crucial data from machines and equipment in real-time. These sensors continuously monitor various parameters such as temperature, vibration, and pressure, offering granular insights into operational performance. The data collected by IoT sensors form the bedrock for further analysis, enabling early detection of potential issues.
Machine learning algorithms play a pivotal role in analyzing the data harvested by IoT sensors. By leveraging historical data and current metrics, these algorithms identify patterns and anomalies indicative of potential equipment failures. For instance, a machine learning model can predict bearing failures in motors by detecting slight deviations in vibration signatures, thus prompting timely maintenance actions. This predictive capability minimizes downtime and extends the life of industrial assets.
Cloud computing also serves an essential purpose in the predictive maintenance ecosystem. It provides a robust infrastructure for storing and processing large volumes of data generated by connected devices. Cloud platforms facilitate centralized data analytics, enabling organizations to cultivate actionable insights from dispersed data points. Solutions like Microsoft Azure and AWS IoT Analytics exemplify cloud-powered predictive maintenance platforms that enhance data accessibility and computational power.
Complementing cloud solutions, edge computing addresses the need for real-time data processing by performing analytics closer to the source of data generation. This approach reduces latency and bandwidth usage, which is particularly beneficial for industries requiring immediate response times, such as manufacturing and energy. Hardware like edge servers and specialized gateways, equipped with powerful processors, execute predictive maintenance algorithms locally, enabling prompt decision-making and reducing the risk of system failures.
Together, these technologies form a cohesive framework that supports the predictive maintenance paradigm. IoT sensors capture critical operational data, machine learning algorithms analyze these datasets for predictive insights, and cloud and edge computing infrastructures process the information efficiently. The synergy between these technologies is pivotal for achieving proactive maintenance strategies in Industry 4.0, ensuring enhanced operational efficiency and reliability.
Benefits of Predictive Maintenance in Industrial Operations
Predictive maintenance has emerged as a pivotal component in optimizing industrial operations, especially within the framework of Industry 4.0. First and foremost, predictive maintenance significantly reduces downtime. By utilizing advanced technologies such as digital twins, machine learning, and sensor data, industrial operations can predict equipment failures before they occur. This anticipation allows for timely maintenance, minimizing unplanned interruptions that can halt production.
Another paramount advantage is the extension of equipment life. Predictive maintenance strategies enable continuous monitoring and analysis of machinery conditions. This approach ensures that maintenance activities are performed based on the actual wear and tear of equipment rather than on a preset schedule. Such precision maintenance preserves the functional integrity of machinery, thereby prolonging their useful life.
Moreover, predictive maintenance fosters enhanced operational efficiency. When industrial operations leverage technologies like digital twins to simulate and optimize maintenance tasks, they can allocate resources more effectively and streamline workflows. This efficient resource management results in higher productivity levels and fewer delays due to equipment malfunctions.
Cost reduction is another notable benefit associated with predictive maintenance. Traditional reactive maintenance often leads to substantial costs arising from unexpected breakdowns and emergency repairs. In contrast, predictive maintenance curtails these expenses by facilitating planned interventions. The deployment of predictive analytics and digital twins helps identify potential issues at an early stage, allowing for cost-effective solutions that prevent costly failures.
Real-world examples underscore these advantages. For instance, a study by McKinsey found that predictive maintenance can reduce downtime by 30% to 50% and lower maintenance costs by 10% to 40%. An automotive manufacturing plant implementing digital twin technology reported a 15% increase in operational efficiency and a 20% reduction in maintenance costs within the first year.
In summary, the adoption of predictive maintenance in industrial operations brings forth a multitude of benefits, including minimized downtime, extended equipment lifespan, heightened operational efficiency, and reduced maintenance expenditures. The integration of advanced technologies like digital twins amplifies these advantages, making predictive maintenance an indispensable strategy for modern industrial settings.
Challenges and Limitations
While the adoption of predictive maintenance heralds numerous benefits, it also introduces several challenges and limitations that industries must address. One of the primary obstacles is the initial cost of implementation. The deployment of predictive maintenance systems can be capital-intensive, encompassing the procurement of advanced sensors, analytical software, and the integration of digital twins. These high upfront costs can be a deterrent for small and medium-sized enterprises (SMEs) with limited financial resources.
Another significant challenge lies in the need for skilled personnel. The effective operation of predictive maintenance systems requires expertise in data analysis, machine learning, and the understanding of digital twins. The scarcity of qualified professionals can hamper the seamless execution of these systems. Industries must invest in training and development programs to bridge this skill gap, fostering a workforce proficient in emerging technologies.
Data privacy and security concerns also pose notable challenges. Predictive maintenance relies heavily on the continuous collection and analysis of vast amounts of data from machinery and equipment. This accumulation of sensitive operational data heightens the risk of cyberattacks and data breaches. Implementing robust cybersecurity measures and establishing comprehensive data governance frameworks are essential strategies to safeguard this information and maintain trust among stakeholders.
The integration of predictive maintenance systems with existing infrastructure presents another hurdle. Many industries operate with legacy systems and machinery that may not be readily compatible with modern predictive maintenance technologies. Retrofitting or upgrading these systems to support the implementation of digital twins can be technically challenging and resource-intensive. Developing scalable solutions and phased integration approaches can help mitigate these difficulties, ensuring smoother transitions and minimizing disruptions to ongoing operations.
In addressing these challenges, industries can consider collaborative efforts with technology providers and academic institutions to innovate and co-create tailored solutions. By fostering an ecosystem of shared knowledge and expertise, the barriers to adopting predictive maintenance can be effectively reduced, paving the way for a more resilient and efficient industrial landscape in the era of Industry 4.0.
Industries Benefiting from Predictive Maintenance
Predictive maintenance, a key component of Industry 4.0, offers extensive benefits across various sectors by utilizing digital twins, advanced analytics, and machine learning. This technology is revolutionizing industries by enabling real-time monitoring and predictive capabilities that drastically improve operational efficiency and reduce downtime. Here we explore how different industries are leveraging predictive maintenance to enhance their operational outcomes.
The manufacturing sector stands out as a primary beneficiary of predictive maintenance. Factories utilize digital twins to create virtual models of their machinery. By monitoring these models, they predict potential failures and optimize maintenance schedules. For example, automotive manufacturers use predictive analytics to monitor machinery wear and tear, significantly reducing unexpected machine failures and production halts.
In the energy sector, predictive maintenance plays a crucial role in ensuring the reliability and efficiency of power generation and distribution systems. Wind farms, for instance, use sensors on turbines to collect data on temperature, vibration, and other critical parameters. By analyzing this data, operators can predict equipment failures and perform maintenance before issues escalate, thus maintaining consistent energy output and reducing operation costs.
Transportation industries also leverage predictive maintenance for enhanced operational efficiency. Railway companies employ digital twins of train components to monitor real-time performance and predict maintenance needs. This ensures the timely replacement of parts, reducing service disruptions and enhancing passenger safety. Similarly, airlines use predictive maintenance to analyze aircraft data, identifying potential technical issues before they lead to costly delays or safety incidents.
The healthcare industry adopts predictive maintenance for medical equipment to ensure the continuous availability and reliability of critical devices. Hospitals use digital twins of medical equipment such as MRI machines and ventilators to monitor their performance. This proactive approach minimizes equipment downtime, thus enhancing patient care quality and operational efficiency.
Through these varied applications, it is evident that predictive maintenance, powered by digital twins and advanced analytics, is fundamentally transforming operations across multiple industries, setting a new standard for efficiency and reliability in Industry 4.0.
Future Trends in Predictive Maintenance
The landscape of predictive maintenance is poised for remarkable transformations owing to the advent of Industry 4.0. One of the foremost emerging technologies leading this revolution is digital twins. By creating a virtual replica of physical assets, digital twins empower businesses to simulate, monitor, and predict potential issues in real-time with unparalleled precision. This integration not only enhances predictive maintenance but also drives efficiency and reduces costly downtimes by addressing maintenance needs proactively.
Complementing digital twins is the evolution of artificial intelligence (AI). Enhanced AI capabilities are ushering in an era where predictive algorithms can analyze vast datasets to identify patterns and anomalies that humans might overlook. AI-driven predictive models can learn from historical data, continuously improving their accuracy in forecasting equipment failures. This means that organizations can preemptively address potential issues based on data-driven insights rather than reactive maintenance protocols, significantly optimizing operational workflows.
Advanced analytics play a crucial role in this transformation. The integration of predictive analytics with IoT sensors and other data collection methods allows for the aggregation of real-time data from various sources. This comprehensive data flow enables deeper insights into equipment health and performance, facilitating smarter decision-making processes. As advanced analytics evolve, they are expected to incorporate more sophisticated algorithms and machine learning techniques, resulting in even more precise and actionable maintenance strategies.
As these technologies continue to mature, the predictive maintenance landscape will witness a further shift towards predictive and prescriptive maintenance. This shift will not only enhance the reliability and lifespan of industrial assets but will also contribute to significant cost savings by minimizing unplanned downtimes and maintenance-related expenses. In essence, the future trends in predictive maintenance, spearheaded by digital twins, advanced AI, and sophisticated analytics, will redefine industrial operations, setting new benchmarks for efficiency and productivity.
Implementation Strategies for Predictive Maintenance
Implementing predictive maintenance within an organization requires a methodical approach encompassing several critical steps. Initially, thorough planning and assessment are imperative. Organizations must first analyze their existing maintenance processes and identify areas where predictive maintenance can offer substantial benefits. This includes conducting a feasibility study that examines technical, operational, and financial aspects, ensuring alignment with the company’s strategic objectives.
Subsequent to planning, the selection of appropriate technology plays a pivotal role. Companies need to evaluate and choose predictive maintenance tools that best fit their operational needs. This may include leveraging advanced analytics, IoT sensors, and digital twins. Digital twins, in particular, offer a virtual representation of physical assets, enabling real-time monitoring and predictive analytics to foresee potential failures before they occur. The integration of such technologies should be seamless, ensuring minimal disruption to ongoing operations.
Training personnel is another cornerstone of a successful implementation. Employees at all levels must gain familiarity with the new systems and understand the underlying principles of predictive maintenance. This can be achieved through comprehensive training programs, workshops, and continuous learning modules. Consistent training not only bolsters the confidence of the workforce but also enhances their ability to leverage predictive maintenance tools effectively.
Continuous monitoring and optimization of the predictive maintenance system are crucial for sustaining its efficacy. Regular audits and performance evaluations should be conducted to identify any potential bottlenecks or inefficiencies. Adopting best practices, such as standardized data collection processes and regular software updates, can help in maintaining the system’s reliability. Moreover, incorporating feedback loops from system users can drive ongoing improvements and innovations.
Best practices to ensure a smooth transition include starting with a pilot project to demonstrate value before full-scale deployment and fostering a culture that embraces technological adoption. By meticulously planning, selecting the right technologies, equipping personnel with necessary skills, and perpetually refining the system, organizations can achieve a successful and sustained implementation of predictive maintenance in the era of Industry 4.0.
Conclusion: The Road Ahead
As we navigate through the transformative landscape of Industry 4.0, the adoption of predictive maintenance emerges as a quintessential strategy for industrial advancement. Throughout this blog post, we have dissected the multifaceted aspects of predictive maintenance, emphasizing its pivotal role in minimizing unplanned downtimes, optimizing resource utilization, and enhancing overall operational efficiency. The advent of digital twins, machine learning algorithms, and IoT technologies facilitates a more profound and accurate forecasting of equipment health, thereby revolutionizing maintenance paradigms.
The integration of digital twins enables organizations to create highly detailed virtual models of physical assets. By leveraging real-time data and advanced analytics, these models offer unparalleled insights into asset behavior and potential failure points, significantly bolstering predictive capabilities. Predictive maintenance driven by digital twins not only mitigates risk but also contributes to cost savings and extended asset lifecycle.
Furthermore, machine learning algorithms play an instrumental role in evolving predictive maintenance strategies. By continuously learning from historical data patterns, these algorithms enhance the precision of predictions, providing actionable insights that are crucial for preemptive interventions. The synergy between IoT sensors and machine learning has unlocked new dimensions in predictive maintenance, making it an indispensable tool in the smart factory ecosystem.
Looking ahead, the future of predictive maintenance in Industry 4.0 appears both promising and imperative. As competitive pressures mount and technological advancements accelerate, organizations that fail to incorporate predictive maintenance risk falling behind. The shift towards a predictive maintenance model not only supports sustainability and efficiency but also aligns with the broader objectives of Industry 4.0, fostering innovation and resilience.
We encourage industry leaders and stakeholders to initiate their journey towards predictive maintenance. Embracing these advanced technologies can significantly enhance competitive advantage and operational excellence. To further explore the vast potential of predictive maintenance, consider delving into additional resources and expert consultations. Your proactive steps in adopting predictive maintenance today will pave the way for a more resilient and efficient tomorrow in the realm of Industry 4.0.